Grouping SaaS cohorts correctly can take a lot of time, but it can be extremely valuable in diagnosing hidden issues which are impacting SaaS customer retention.
As an analogy, we have several fruit trees on our land in Kansas. Some do well most every year. Others rarely produce. Trying to diagnose the issues and improve our harvest is like making a jigsaw puzzle without a picture. Much like diagnosing a SaaS business, I tend to tackle our fruit trees in cohorts.
The first most obvious is to compare production by type of fruit; apples, pears, peaches, and so forth. This would be like comparing SaaS customers by plan type; Personal, Standard, Business, and Enterprise. This is always a great way to get started with a cohort analysis. The retention data is usually available, and the results typically yield valuable information. The answers are somewhat obvious too though. Pears are more drought resistant. Peaches and Nectarines are favorites of birds and bugs. Personal and Standard plans tend to churn more. Enterprise plans have higher net dollar retention rates.
To really learn what is going on and make the necessary changes we need to dig deeper. With the trees other considerations include cohorts such as;
- Which trees were planted in the fall versus the summer?
- Which trees are on the east side of the orchard versus the west?
- Were the trees planted before or after the 2012 to 2014 drought years?
- In what year were the trees planted?
That last one tells us a lot. The year the trees were planted. Looking at cohorts by year can tell us why they are doing well, or not. To start with it gives us an age, which is always valuable. It also allows us to reflect on other issues throughout those years like the droughts, or that terrible year the young deer bucks found their way into our orchard and nearly destroyed several trees when rubbing their velvet antlers.
A “Normal” Example
Let’s take a look at what a SaaS cohort analysis could look like when comparing customers by their start dates and grouping by year. The next chart is comparing logo count over time. It is a normalized chart with retention and new customer creating a linear logo count growth. Not ideal, but easy to understand for demonstration. Each year more logos are added and each year there is churn as can be seen with each year trailing downward over time. While this analysis is using logo count, it is more common to use a revenue metric. Both gross and net dollar can be used, but I prefer logo count as a starting point.

Unfortunately, in this normalized cohort analysis chart, there isn’t really a whole lot to learn. If this is how the first pass analysis of a logo count cohort looked, it would be an uneventful discussion when sharing the results with management.
A normal SaaS cohort analysis by year will expose both strengths and weaknesses across the value chain of product, marketing, sales, customer success, and even IT infrastructure. Any anomalies identified could bring up considerations like;
- Was there substantial product functionality added in any specific years?
- Which marketing campaigns were driving traffic during strong or weak years?
- Who were the lead sales people during peaks and valleys?
- How were NPS or CSAT scores during years with anomalies?
- Were there infrastructure issues such as latency or downtime?
A More “Realistic” Example
Now let’s look at a more realistic example of a SaaS cohort analysis by year where there is indeed an issue to address. In the following example you will see significant retention issue with customers acquired during the first half of 2019.

At first glance this chart looks nearly identical to the first. Afterall, it is exactly the same data as before with only one exception. Throughout the year of 2020 the retention rate for the 2019 cohort (grey in color) takes a nosedive. An immediate reaction is to look at customer success or account management during the year of 2020 as the issue. What was going on to allow the retention rates in 2020 to slide?
However, 2020 customer success or account management is probably not the area for the root cause. By digging deeper we see that no other cohort in the calendar year of 2020 was having a retention issue beyond the normal. It was only the customers acquired during the first half of 2019. A few more likely scenarios could include;
- How long was the onboarding process for 1H 2019 compared to other cohorts?
- Were different sales strategies employed during the 1H 2019 closing period that could have set unique customer expectations?
- Did the marketing source change significantly for 1H 2019 (paid search, organic, social, etc.)?
Top 10 Cohorts for Analysis
After the first pass at the customer cohort by acquisition year, we see the need to peel back more layers. In order to properly diagnose the issue, and more importantly learn and correct this issue, we must slice and dice the data again. Here are the top 10 most common SaaS cohorts used for analysis.
- Initial plan type
- Year acquired
- Revenue (MRR or ARR), could also be tied to user count or plan type
- Marketing source
- Assigned CSM or implementation personnel
- Industry, vertical, or sector
- Customer size (SMB, Mid-market, Enterprise, employee count, user count)
- Customer location
- Time to onboard (acquisition date to definition of “live” date)
- Time of year (sector seasonality issues)
With access to data, there could be many more than listed here. Cross referencing one against the other is useful too. For instance, knowing the marketing source and comparing that to the industry or sector can tell us a lot about the effectiveness of our marketing strategies. Another example would be comparing the time to onboard to the company size. This could expose a strength or weakness in the team’s ability to navigate enterprise customers versus solopreneurs.
In Summary
In our original analogy of our Kansas fruit orchard, it is fundamental to a successful analysis that we have accurate data on what type of trees were planted and when. We keep a journal, but much like SaaS business metrics, we are missing data. In hindsight we would like to know where we bought the trees, the type of nutrients used when planting, and any maintenance records along the life of the tree.
When analyzing a SaaS business, it is sometimes challenging to recreate data like the marketing source, the implementation personnel, or sales rep closing the deal. The better records we keep, the faster, easier, and more accurate our cohort diagnosis. Of course, the real key to success is to learn from the diagnosis and take corrective action.
Photo credit to Petra Hucht
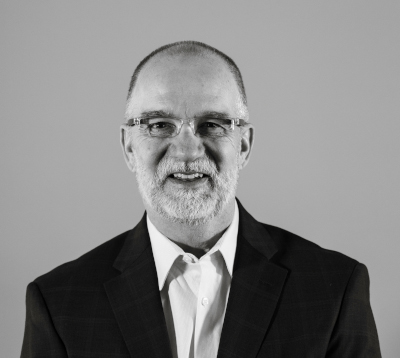
If you need help thinking through this or other leadership challenges, let’s have a discussion to see if I can help in some way.